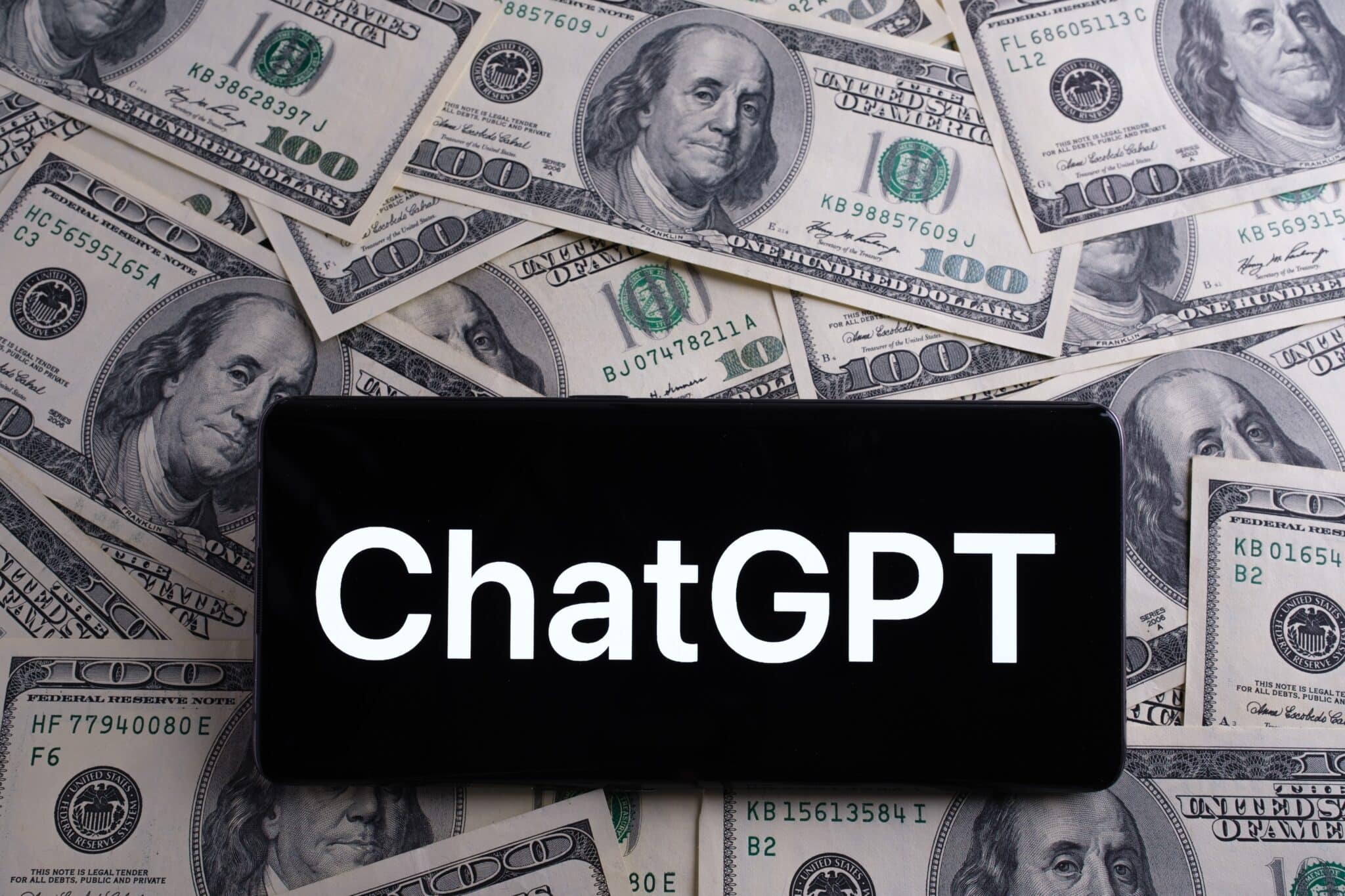
This article was originally published in Food Logistics.
Over the first quarter of 2023, you’ve undoubtedly seen headlines regarding a new breed of artificial intelligence (AI) tools and chatbots, known as large language models (LLMs), which have the potential to augment or transform how we learn, live and work. For starters, ChatGPT by OpenAI took the world by storm with its almost-magical ability to respond to nearly any request with a detailed and informed reply. Since then, the world’s tech giants and the open-source community have rapidly produced their own advanced products, plug-ins or integrations to accelerate this new AI revolution.
Those in supply chain logistics have been through technological upheavals in the past: the introduction of EDI and other instant data transfers, the IoT (Internet of Things) giving real-time tracking and telemetry data, cloud computing providing massive data stores for extensive data analysis and SaaS (software as a service) offerings, and the creation of AI and automation tools to maximize efficiency.
So, what exactly are LLMs, and how will they transform logistics supply chain operations now and in the future?
What is a large language model?
Known as command-and-control systems, these bots can understand and respond to a finite (albeit large) list of questions and requests they have been programmed to process. These short prompts are processed individually, one at a time, and have little to no connection to previous requests.
ChatGPT by OpenAI, Google Bard, and open-source projects are advanced LLMs. Trained on vast amounts of data, LLMs are designed to understand and generate conversational text on virtually any subject. Large language models like ChatGPT work by processing a request and then predicting the best possible words or phrases to respond with, word by word, until the request is fulfilled, making it an ideal tool for facilitating communication, complex problem-solving and providing valuable insights and recommendations.
LLMs can respond to extremely long, complex, multi-part questions and retain knowledge of previous conversations to build from. From the outset, its business applications are both powerful and versatile: it can write and debug computer programs, translate and summarize text, develop and document business pitches, craft marketing plans and social media campaigns, and provide analysis and insight on nearly any subject it has been trained on.
A challenge for ChatGPT and other LLMs is that the systems are trained on vast amounts of generally accessible data up to a given date. In the case of ChatGPT, that date is late 2021. This means responses regarding topics without new advances or information since that end date could return inaccurate or outdated results. In recent months, most LLMs have added access to query live resources on the internet and created the ability for developed plug-ins and extensions to enhance a model’s capabilities through other datasets (including ways to utilize your own proprietary data safely). For very sensitive data, many commercial and open-source LLMs can be downloaded and run privately within a secure network.
How LLMs can improve supply chain operations
There are many ways in which these systems can help streamline logistics operations and overall supply chain performance.
Enhanced demand forecasting and inventory management accurate demand forecasting ensures an efficient and optimized supply chain. LLMs can analyze vast datasets, including consumer behavior, market trends and historical sales data. By incorporating such information and additional data points such as point-of-sale systems, social media and weather patterns, these models can provide more accurate predictions on consumer demand, enabling your company to streamline their production and distribution processes, reduce carrying costs and enhance customer satisfaction.
Improve on-time in-full delivery
LLMs are a fantastic problem-solving tool, simplifying complex insights and KPIs by giving easy-to-understand context around those metrics. For example, it can look at data and identify problem areas within operations, narrowing down the root causes of most issues.
It can then provide creative solutions to these problems, like suggesting changes to pick-up or delivery dates. These systems can also be used to consolidate loads. It would choose the most optimized route not solely based on complex data such as delivery due date but also considering location, type of shipment and the consistency of capacity, as well as externally accessible information, such as weather, traffic, port congestion and more.
This is excellent news for consumer packaged goods (CPG) shippers who must meet strict must-arrive-by-dates (MABDs) or a specific on-time, in-full delivery (OTIF) score to comply with major retailers.
LLMs can also make intelligent pricing estimates by considering factors like a standard pricing model, market conditions, news and history. It could also break down rates by certain months and make it easy to cross-compare current rates vs. historical ones. Given access to freight data, the system could help identify potentially problematic shipping locations, predict additional accessorial charges and more.
Brands can immediately leverage LLMs to analyze data in natural language, gaining insights to improve day-to-day logistics operations. Using transformers that have studied a data set or by augmenting AI, you can better understand the complexity of probable movement progression and spot warning signs proactively. This also enables chatbots to speak intelligently to the nuances of unique customers. For example, it could communicate load details to a specific customer just by being asked naturally and understanding the response styles that the customer is most comfortable with.
The bot can evaluate multiple notes in an order and summarize them. For example, “Due to weather conditions and changes in the customer’s pickup, the original arrival date of this order changed.” It adds more of a human element to automated interfaces and communications as well, easily composing and delivering email updates regarding the status of shipments.
LLMs can be extended to perform actions such as appointment scheduling on your behalf via third-party APIs, EDI or other communication methods. They can also integrate with other parts of an organization’s technology stack, like robotic process automation systems, which can log into retailer portals to review availability, make suggestions, and book preferred dates and times.
Overall Impact of LLMs
Integrating LLMs for the CPG logistics supply chain holds immense potential for transforming the industry. These models empower businesses to make data-driven decisions, enhance efficiency, reduce costs and improve overall customer satisfaction.
The pace of innovation in the last six months alone is staggering. Businesses that choose to embrace this evolution will be well-positioned to be competitive, flexible, and sustainable in the coming years. Take the first step in completely transforming supply chain decision-making by exploring these AI services and ask these systems to give insight into a current focus point within your operations.
KanoPI: Zipline Logistics’ Proprietary Shipping Intelligence Tool
Built in-house by Dustin Verdin – the author of this article – and improved daily by the Zipline Development Team, KanoPI is our proprietary shipper intelligence tool. Our retail logistics experts leverage KanoPI to optimize client supply chain efficiency, reduce costs, and skyrocket logistics performance.
KanoPI has helped Zipline clients achieve up to:
- $1.2 Million in transportation savings
- 20% reduction in cost per pallet through consolidation
- 17% improvement in meeting on-time in-full delivery (OTIF)
- 30% increase in network efficiency
- 861,908 fewer projected miles traveled overall
Our technology suite offers shippers with revenue between $1 Million and $1 Billion the visibility and insight “Big Box” 3PLs reserve for Fortune 100 customers.
KanoPI provides Zipline clients with:
- High-powered visibility and data to track and trace shipments
- Weather overlays and proactive alerts of potential disruptions
- Reports on logistics performance and cost analyses to make timely, cost-effective decisions
- Queries and sorts data so users can identify new opportunities